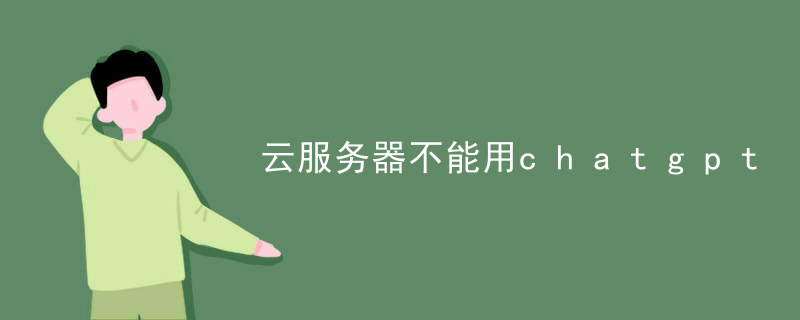
Cloud server cannot use chatgpt
Cloud servers cannot use ChatGPT
Cloud servers have become the preferred choice for many enterprises and individuals, and have achieved widespread applications. Although cloud servers have significant advantages in providing efficient computing and storage resources, they are not suitable for running artificial intelligence models such as ChatGPT. Below, we will elaborate on this viewpoint from three aspects: technology, resources, and security.
ChatGPT is a large-scale language model based on deep learning, with a large scale and numerous parameters. In order to run ChatGPT, many computing resources and memory space are required to support the training and inference process of the model. However, the computing power and storage resources of cloud servers are limited, making it difficult to meet the needs of large-scale models like ChatGPT. Even though cloud servers themselves can support high-performance computing, the resource requirements required to run ChatGPT still far exceed the capabilities of cloud servers.
Although cloud servers have high computing power, they have not been optimized for models like ChatGPT. ChatGPT is a model that requires a large amount of computation and requires high performance of the server's CPU and GPU, while cloud servers are often more suitable for handling general computing tasks and cannot meet the specific hardware requirements of ChatGPT. Even though cloud servers can adjust their configuration to meet a certain level of demand, due to their inherent architecture limitations, they cannot provide the optimal performance required by ChatGPT.
Security is another key issue in running ChatGPT. ChatGPT is a powerful language model that can generate various human languages, requiring access to a large amount of training data and model parameters. If ChatGPT is run on a cloud server, it is necessary to store these sensitive data in the cloud, which poses a risk of data leakage. Cloud servers are usually maintained and managed by cloud service providers, and users cannot fully grasp and monitor the security of cloud servers, which also poses hidden dangers to the deployment of ChatGPT.
Although cloud servers have significant advantages in providing computing and storage resources, they are not suitable for running large language models such as ChatGPT. Due to limitations in computing resources and memory space, cloud servers are unable to meet the resource requirements of ChatGPT; The cloud server is not optimized for ChatGPT and cannot provide optimal performance; Due to security considerations, deploying ChatGPT on cloud servers may lead to data leakage and security risks. When choosing a suitable computing platform, we need to comprehensively consider the requirements of the model and the limitations of cloud servers to find the best solution.
-
1
Chatgpt Deploying Cloud Services
Chatbot has become the preferred method for many enterprises and developers to deploy cloud services. With the continuous development of artificial intelligence technology, Chatbot is becoming an important communication method between enterprises and customers. In the past, building and deploying Chatbot may require a significant amount of resources and complex technical knowledge. Using a cloud service provider's platform, we can quickly and easily deploy Chatbot to the cloud. Deploying Chatbot to cloud services has many advantages. Cloud services provide powerful computing and storage capabilities, which enable Chatbot to handle a large number of user requests and data. As the number of users increases, we can easily adjust the scale of cloud services to meet demand. Cloud service providers typically have high availability and redundancy mechanisms, which ensure that Chatbot remains online at all times. Cloud services typically also provide security and backup capabilities to ensure the protection of Chatbot data and user information. The process of deploying Chatbot to cloud services is relatively simple. We need to choose a cloud service provider, such as Amazon AWS, Microsoft Azure, or Google Cloud Platform. These platforms all provide specialized tools and services for deploying Chatbot. We need to create a cloud server instance and configure the operating system and network settings. We need to install the running environment for Chatbot, such as Python or Node.js. Once the environment is set up, we can upload the code or model of Chatbot to the cloud server for installation and configuration. We can use the management console or API of cloud service providers to start and monitor the running status of Chatbot. After deploying Chatbot to cloud services, we can easily integrate and expand with it. By using APIs and tools from cloud service providers, we can connect Chatbot with other services and systems, such as CRM systems, email services, or third-party APIs. This enables Chatbot to obtain more information and data to provide more accurate and personalized answers. Cloud services typically provide developer friendly interfaces and documentation, making it easier for us to customize and modify. NN cloud service providers typically provide flexible billing methods. We can choose different billing modes based on actual usage, such as charging based on usage time, request volume, or subscription method. This enables us to manage and control costs reasonably based on budget and requirements. When deploying Chatbot to cloud services, we also need to pay attention to some considerations. We need to ensure that the configuration and performance of the cloud server can meet the needs of Chatbot. If the number of requests processed by Chatbot is very large, we may need to choose high-performance instance types or use load balancing to improve processing capabilities. We need to consider the security and privacy protection of data. Cloud service providers typically provide some security measures, such as data encryption and authentication. We should choose appropriate security settings based on actual needs. We also need to regularly monitor and maintain the operational status of cloud services and Chatbot to ensure their normal operation and performance optimization. Deploying Chatbot to cloud services is a fast, flexible, and reliable method. Cloud services provide powerful computing power, high availability, and security, making it easy for us to build and deploy Chatbots. By integrating with other services and systems, we can provide users with a more comprehensive and personalized experience. During the deployment process, we need to pay attention to some technical and security issues. Only through reasonable planning and management can we fully utilize the advantages of cloud services and provide efficient and high-quality Chatbot services.
-
2
Chatgpt proxy server
Chatbot is an artificial intelligence technology that can simulate human conversations and provide the ability to ask and answer questions. Chat robots have attracted widespread attention in various fields. One common use is to combine chatbots with proxy servers to form a technology called "ChatGPT proxy server". The nnChatGPT proxy server is a chat robot proxy server based on the OpenAI GPT model (Generic Pre trained Transformer). The GPT model is a powerful artificial intelligence model that can generate coherent text and responses. By combining the GPT model with a proxy server, we can achieve large-scale chat robot services and provide users with a rich interactive experience. The workflow of the nnChatGPT proxy server is as follows: Users send requests to the proxy server through the client, which can include questions, instructions, or other content that needs to be processed. After the proxy server receives the request, it passes it on to the GPT model for processing. The GPT model will analyze the request content and generate corresponding responses or execute corresponding instructions. The proxy server sends the generated answer back to the user. The application scenarios of nnChatGPT proxy servers are very extensive. It can be applied in the field of customer service. By deploying the ChatGPT proxy server on the customer service platform, users can have conversations with chat robots through online chat windows, ask questions, and receive answers. This greatly improves the efficiency of customer service and can provide services to multiple users simultaneously. The nnChatGPT proxy server can also be applied in the field of virtual assistants. Virtual assistants are artificial intelligence applications that can simulate human assistants. By combining with ChatGPT proxy servers, virtual assistants can engage in more intelligent conversations and provide more personalized and customized services. Virtual assistants can recommend movies, music, or tourist attractions to users based on their preferences and needs. The nnChatGPT proxy server can also be applied in the field of online education. Online education platforms can use ChatGPT proxy servers to provide online Q&A services for students. Students can ask questions through dialogue and receive detailed answers. In this way, students do not need to wait for the teacher's response and can receive help and guidance at any time. The nnChatGPT proxy server also has some challenges and limitations. The training of the GPT model requires a large amount of data and computational resources. Due to the large number of model parameters, high-performance hardware is required for training and inference. GPT models may sometimes generate inaccurate or unreasonable answers. This requires us to incorporate necessary limitations and constraints when designing and training the model to ensure that the generated responses comply with semantics and logic. The nnChatGPT proxy server is a chat robot proxy server based on the GPT model. It can be applied in fields such as customer service, virtual assistants, and online education, providing intelligent dialogue and Q&A functions. Although there are some challenges and limitations, with the development of artificial intelligence technology, ChatGPT proxy servers will play a greater role in the future.
-
3
Chatgpt Deploying Cloud Server
ChatGPT is an open source model developed by OpenAI for natural language processing tasks. It is an artificial intelligence model based on deep learning that can be applied in various fields, such as customer service, virtual assistants, etc. In order to use ChatGPT in a production environment, we need to deploy it to a cloud server. Cloud servers can provide powerful computing power and flexible deployment methods, enabling us to better interact with ChatGPT. We need to choose a cloud service provider, such as Amazon Web Services (AWS) or Microsoft Azure. These service providers provide a series of virtual machine instances that can be used to deploy our ChatGPT model. We need to select an instance type with sufficient computing resources. This depends on our desired performance and budget. Usually, GPU instances are more suitable for deep learning tasks than CPU instances because they can provide faster training and inference speeds. We need to create a virtual machine instance and select an operating system. In most cases, we can choose a Linux operating system, such as Ubuntu or Amazon Linux. These operating systems have extensive support and documentation, and are very suitable for deploying deep learning models. On the virtual machine instance, we need to install some necessary software. We need to install the Python environment and related deep learning libraries, such as TensorFlow or PyTorch. These libraries can help us load and run the ChatGPT model. We need to upload the ChatGPT model and related code to the virtual machine instance. We can use Git or other file transfer tools to complete this task. The model file may be large, so we need to ensure that the virtual machine instance has sufficient storage space. Once the file upload is completed, we can start configuring the server. We need to write a startup script to start the ChatGPT model and the applications that interact with it. This script can be customized according to actual needs, such as configuring network ports, loading model files, etc. We need to start the server and conduct some testing. We can use command-line tools or write a simple client application to interact with ChatGPT. We can connect to the cloud server through the network and have real-time conversations with ChatGPT. This is just a simple deployment process example. Deploying the ChatGPT model to cloud servers also involves many other aspects, such as security, performance optimization, and automation. However, through the above steps, we can preliminarily achieve a fully functional ChatGPT deployment. Deploying ChatGPT to cloud servers is a complex process that requires a certain level of professional knowledge and skills. However, through reasonable configuration and optimization, we can fully utilize the computing power of cloud servers to provide users with high-performance and intelligent chat experiences.
-
4
The server used by chatgpt
The server nnChatGPT uses is a powerful natural language processing model based on artificial intelligence technology, which can perform tasks such as automatic question answering and dialogue. In order to ensure the efficient operation of ChatGPT, it requires strong server support. The server used by nnChatGPT is mainly divided into two parts: training server and inference server. The training server is used for the training process of the model, while the inference server is used for practical applications and interactions. The NN training server is the cornerstone of ChatGPT. During the training process, ChatGPT requires a large amount of computing resources and storage space. To meet this requirement, training servers are usually equipped with multiple high-performance graphics processors (GPUs) or tensor processors (TPUs), which can accelerate the computation speed of matrix operations and deep learning algorithms. The NN training server also needs sufficient memory space to store model parameters and intermediate calculation results. The model parameters of ChatGPT are usually very large, with hundreds or even billions of parameters. Training servers are usually equipped with large capacity random access memory (RAM) to ensure that model parameters can be fully loaded and manipulated. The NN training server also requires a high-speed network connection to download large-scale training datasets from the cloud or other data sources. These training datasets typically include billions of question and answer pairs, conversation records, etc., used for model learning and optimization. A fast and stable network connection can improve the efficiency of data transmission, thereby accelerating the training process. On the other hand, inference servers are used for practical applications and interactions. When a user uses ChatGPT for questioning or dialogue, the inference server is responsible for responding to the user's request and generating corresponding answers. Compared to training servers, inference servers have relatively lower requirements. Due to the fact that the inference process only involves forward computation and does not require backpropagation or gradient updates, the demand for computing resources is relatively small. NN inference servers are usually equipped with high-performance central processing units (CPUs) and sufficient memory space to ensure fast processing of user requests. The inference server also requires a stable network connection in order to interact with users in real-time. In order to ensure the high availability and load balancing of ChatGPT, NN usually configures multiple inference servers and distributes user requests to different servers through load balancing technology. This can improve the overall performance and reliability of the system, ensuring that users can quickly obtain satisfactory answers. The server used by nnChatGPT has different requirements in the training and inference stages. The training server requires strong computing and storage capabilities for training and optimizing model parameters; The inference server requires fast response speed and stable network connection for practical applications and interactions. By properly configuring the hardware and software resources of the server, the performance and user experience of ChatGPT can be improved.
Popular Services
More-
AI customer service
Fully automated intelligent assistant, making services smarter and more efficient